By Tian Wang, Ph.D and Navid Sadeghi, Ph.D.
Martensitic grains are hard to analyze
Martensite, one of the hardest steel structures, is formed when austenite is rapidly quenched to a low temperature. This prevents carbon diffusion and formation of pearlite or bainite [1] but creates thin plates or lath morphologies [2]. Specific characteristics of this microstructure makes optical analysis of martensitic grains challenging.
Martensitic grains become finer with increasing carbon content, changing from lath to plate morphology [3]. However, this reduces distinctive structural shapes, forcing practitioners to measure the size of the prior austenite grains to interpret the martensitic transformation and optimize the heat treatment conditions [3]. In addition, in low-carbon steels the etching procedure cannot reveal prior austenite boundaries very well, leading to labs often relying on the human eye to distinguish and classify martensitic grains.
To make matters worse, the common thresholding technique used in automated image analysis software is unsatisfactory for this kind of image (Fig. 1). In gray level thresholding, we use the distribution of pixel values in a black and white image and select a pixel value, or threshold, to optimally separate two groups of pixels. This method therefore works best with clearly defined phases that form separable peaks in the distribution of pixel values. But in martensitic surfaces, the gray levels may be distributed without distinct peaks (Fig. 1a), or grains may contain a mixture of both groups of pixels. This makes thresholding ineffective for partitioning, or segmenting, the image into grain regions of interest (Fig. 1b).
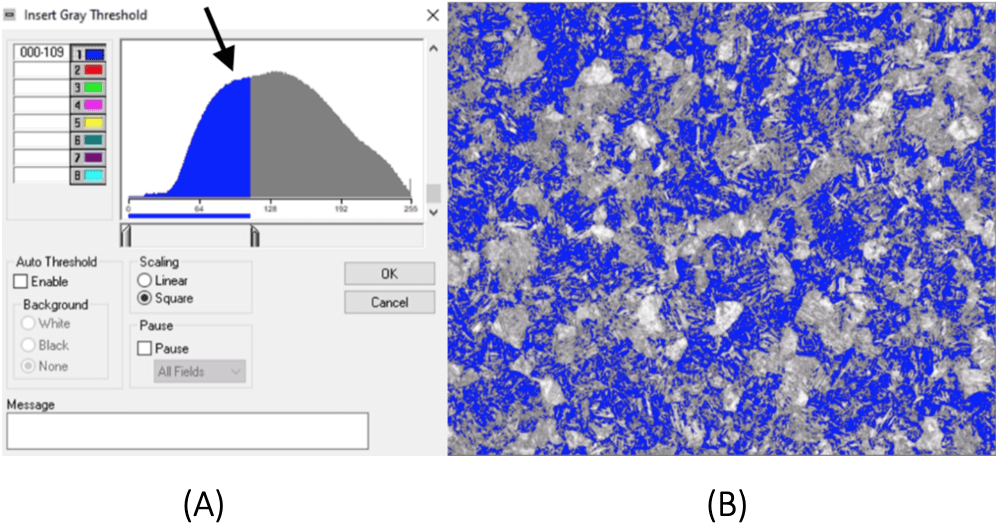
Figure 1. Grayscale thresholding method.
(A) No distinct peaks or valleys can be seen from the histogram (arrow) of the grayscale image.
(B) The resulting segmentation using thresholding (blue) cannot be used in automated analysis routines for measuring grain size.
A new method for martensitic analysis
Clemex’s Vision PE now has an automatic way of processing images of various martensitic structures that does not depend on gray level thresholding (Fig. 2). This new method uses recent machine learning algorithms and image processing techniques to rapidly and accurately identify martensitic grains.
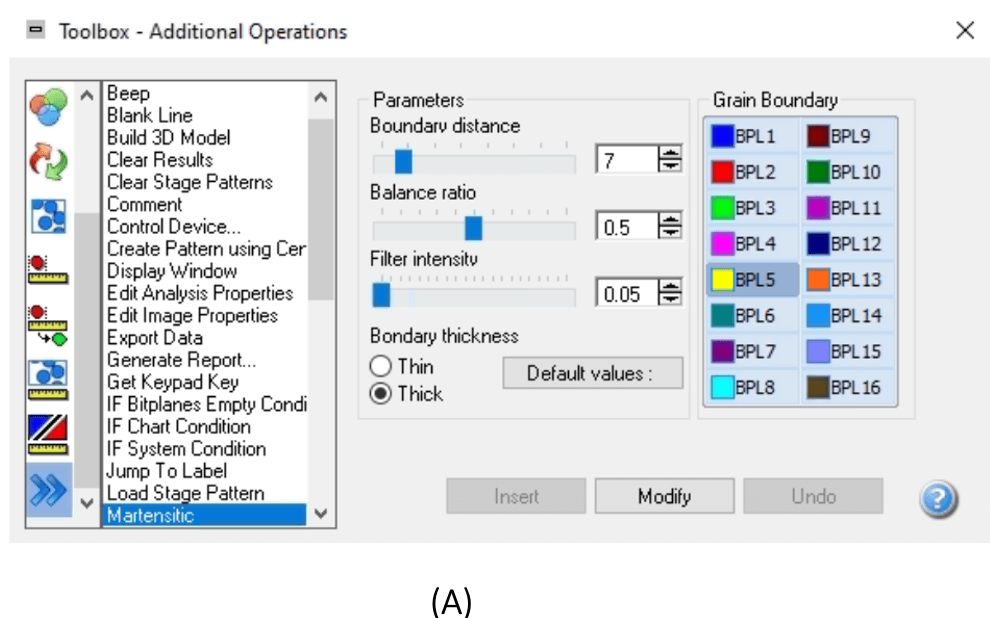
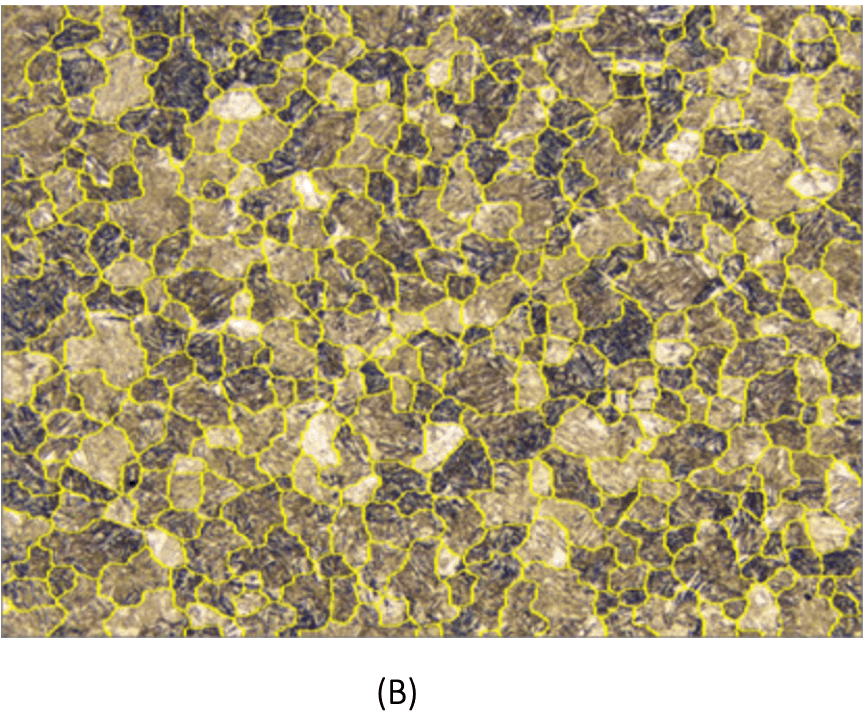
Figure 2. New martensitic algorithm
(A) The powerful Martensitic functionality is inserted at the beginning of an automated analysis routine, and can be fine-tuned usually by adjusting just one parameter.
(B) The size of the detected grains (yellow) is then measured automatically and a report is generated according to ASTM E-112.
The special properties of martensitic microstructure make their grains look different from each other and across different images. Our algorithm works out of the box, without requiring labor-intensive annotation of these variable grains or a priori knowledge about their types and number. The method is hence not specific to a special type of surface and can be successfully applied to different martensitic structures by adjusting a few parameters This also means that the method can track any unexpected microstructural changes in the production line from day to day.
The technique is computationally efficient, allowing fast identification of martensitic grains and is therefore high throughput and suitable for real-time analysis. Because of ease of use and freedom from user-dependent criteria such as a threshold, the method minimizes inter-operator variability and increases reproducibility. Our method is also robust to variability in image acquisition factors such as lighting.
Conclusion
The new automatic algorithm implemented in Clemex Vision PE thus provides a fast, general, reliable, and accurate way of optically identifying challenging martensitic grains. This algorithm has been successfully tested on hundreds of images in collaboration with a leading industry partner, and opens up new avenues for demanding analyses of martensitic and similar surfaces, a task that had remained elusive till now.
References
[1] W. D. Callister and D. G. Rethwisch, Materials science and engineering: An introduction, 8th Edition, Wiley Global Education, New York, 2009
[2] H. Bhadeshia and R. Honeycombe, Steels: Microstructure and Properties 4th Edition, Elsevier Ltd, 2017
[3] G.F. Vander Voort, ASM Handbook, Volume 9: Metallography and Microstructures, 2004, pp. 670-700.
Fill in the form below to download a pdf version